April 06, 2022
ARTIFICIAL INTELLIGENCE IS ONE OF THE MOST PROMISING DIRECTIONS OF COMPUTER SCIENCE DEVELOPMENT
In the age of big data society has a need for processing vast amounts of information in real time. That is why the usage of artificial intelligence has become so essential in many areas, including banking and medicine. In this context scientists are entrusted with the significant task of creating a model of a brain, relieving the secrets of thinking and processes of understanding the information. The point at issue is the creation of computer technology and robots with artificial intelligence that can understand, speak and even joke using human speech. At the same time artificial intelligence is considered as a wide-ranging concept that includes intelligent decision-making systems, technologies for creating such systems.
The European Parliament adopted the resolution “Civil Law Rules on Robotics”, which considers various aspects of robotics and artificial intelligence, proposing to establish the legal basis for the usage of the artificial intelligence and introduce a pan-European system for the registration of “smart” machines.
Scientists assert that the existence of artificial intelligence technologies implies the system’s capacity to accumulate, process and apply acquired knowledge and skills, which normally do not require the usage of human intelligence. The main purpose of creating artificial intelligence systems is to help people perform simple operations and routine processes.
History of artificial intelligence systems development
In the early 20th century a generation of scientists, mathematicians and philosophers, who were developing the concept of artificial intelligence, was formed. One of such people was Alan Turing - a young British scientist, who studied mathematical possibilities of artificial intelligence. Alan Turing assumed that if people use available information as well as the ability of their minds to solve diverse problems and make decisions, then why it should be impossible for machines. This was the logical basis for his work “Computing Machinery and Intelligence”, published in 1950, in which the principles of creating intelligent machines and testing their intellectual capabilities were analysed.
However, the number of factors prevented Alan from starting work immediately. First of all, computers had to undergo radical changes, because they did not contain the key prerequisite for intelligence: they could only execute commands, but were unable to store them until 1949. Secondly, calculations were extremely expensive. In the early 20th century, computer leasing cost $ 200 000 a month. Only prestigious universities and large technology companies could afford it.
Five years later, Allen Newell, Cliff Shaw, and Herbert Simon initiated the preparation of the program “The Logic Theorist” in order to implement the concept. “The Logic Theorist” was a program designed to simulate human problem-solving skills and was funded by the research and development corporation (RAND). It is considered to be the first artificial intelligence program. It was presented at the Dartmouth Summer Research Project on Artificial Intelligence (DSRPAI), organised by John McCarthy and Marvin Minsky in 1956. At this historic conference, John McCarthy presented a joint work for open discussion, introducing the term “artificial intelligence” into scientific circulation. The conference became a catalyst for the following 20 years of artificial intelligence research.
In the 1960s, the United States Department of Defence became interested in this type of work and began training computers to imitate basic human considerations. For instance, the Defence Advanced Research Projects Agency (DARPA) completed street mapping projects in the 1970s.
From 1957 to 1974 artificial intelligence developed rapidly, computers were improved: they became faster, cheaper, more affordable and could store more information. Additionally, machine learning algorithms were improved. Newell and Simon's “General Problem Solver” and Joseph Weisenbaum's “ELIZA” showed promising achievement of goals of problem-solving and interpretation of speech, respectively. These achievements, as well as the advocacy of leading researchers (namely DSRPAI members), persuaded governmental institutions, such as the Defense Advanced Research Projects Agency (DARPA), to fund research of artificial intelligence systems in several institutions. The government was particularly interested in a machine that could transcribe and translate speech as well as perform highly productive data processing. “In three to eight years we will have a machine with the general intelligence of an average human being” - this assertion was made by Marvin Minsky in his interview with Life Magazine in 1970.
Nevertheless, the initial plan of development of artificial intelligence faced difficulties. The greatest challenge was the lack of computing power: computers could not store enough information and process it in real time. Hans Moravec, an Massachusetts Institute of Technology doctoral student of John McCarthy stated: “computers were still millions of times too weak to exhibit intelligence”. As no quick results were achieved, funding for research was declining, which caused research to last for decades.
In the 1980s, research in the area of artificial intelligence became more intensive due to the expansion of the set of algorithmic tools and increased funding. John Hopfield and David Rumelhart promoted "deep learning" techniques that allowed computers to learn from their own experiences.
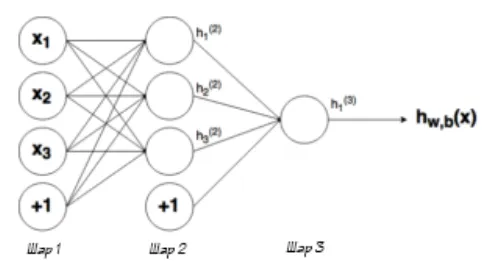
On the other hand, Edward Feigenbaum presented expert systems that imitated the decision-making process of a human, who is an expert. The program asked an expert in a particular field how to react in a given situation, and, as soon as it learned reactions for almost every situation, users were able to get advice from this program. Those expert systems were widely used in industry.
The Japanese government largely funded research of the development of expert systems and other work related to artificial intelligence as a part of Fifth Generation Computers Project (FGCP).
In 1982-1990, $ 400 million was invested in research in order to improve logical programming and artificial intelligence. Funding for the FGCP soon ceased, but the direction of research that involved the development of artificial intelligence was not closed.
During the 1990s - 2000s, a lot of goals set by artificial intelligence researches were achieved. In 1997, speech recognition software developed by Dragon Systems was implemented on Windows. That was another major step towards interpretation of the speech. Even human emotions were reproduced to some extent. The evidence for that was the Kismet robot, developed by Cynthia Brizil, which could recognize and demonstrate emotions.
An obstacle to the expansion of computer storage that had existed for 30 years was overcome. According to Moore's Law, storage capacity and speed of computers doubles every year.
Four types of artificial intelligence
The reactive machine follows the most basic principles of artificial intelligence and, as its name implies, is capable of only using its intelligence to perceive the world around and respond to it. Systems of artificial intelligence, like reactive machines, have low storage capability, and as a result cannot rely on past experiences to inform decision making in real-time.
A famous example of a reactive machine is Deep Blue, which was designed by IBM in the 1990’s as a chess-playing supercomputer and defeated international grandmaster Gary Kasparov in a game. Deep Blue was only capable of identifying the pieces on a chess board and knowing how each of them moves based on the rules of chess, acknowledging each piece’s present position, and determining what the most logical move would be at that moment. The computer was not pursuing future potential moves by its opponent or trying to put its own pieces in better position. Every turn was viewed as its own reality, separate from any other movement that was made beforehand.
Another example of a game-playing reactive machine is Google's AlphaGo. AlphaGo is also incapable of evaluating future moves but relies on its own neural network to evaluate developments of the present game, giving it an edge over Deep Blue in a more complex game. AlphaGo also bested world-class competitors of the game, defeating chess champion Lee Sedol in 2016.
Limited memory artificial intelligence systems have the ability to store data about previous events to predict their development on the basis of gathered information and evaluation of potential decisions. Limited memory artificial intelligence systems have more complex functionality and provide greater possibilities than their predecessors - reactive machines.
Limited memory artificial intelligence systems are used by a team that constantly teaches the model to analyse and utilise new data so that these models can be automatically trained and updated. While using limited memory artificial intelligence systems in machine learning: training data is created; models of machine learning are being developed that have the ability to make predictions, to embed in the model the ability to receive feedback from human beings or the environment, to store data, to reiterate steps as a cycle.
Theory of mind - the ability to recognize the difference between one’s own and someone else’s point of view in order to achieve the next level of development of artificial intelligence systems.
The concept is based on the psychological grounds of understanding the abilities of living beings that affect human behaviour. In terms of artificial intelligence systems it would mean comprehending how humans, animals and other machines feel and make decisions. Artificial intelligence systems gain the ability to perceive and process information, analyse emotions while making decisions and a lot of other psychological reactions in real time, establishing communication between people and artificial intelligence systems.
Artificial intelligence systems based on the theory of mind consist of three artificial neural networks, each of them includes computing elements and connections between them remotely resembling the human brain. The first network analyzes the actions of other artificial intelligence systems and tries to identify certain tendencies in their previous steps. The second one forms an understanding of their “thoughts” and the third one generates a prediction of their behaviour, which is based on the received information.
Self-aware artificial intelligence systems are systems with the highest and most complex level of self-awareness. They are aware of their existence, their inner states, potential emotions; they can form memories of the past and make predictions for the future; they can take into account previous experience in decision making. Self-aware artificial intelligence systems can learn and become smarter from their experience, require extremely flexible programming logic, acquire the ability to update their own logic and tolerance for inconsistencies, given that human behaviour is not always predictable.
The main areas of research in the field of artificial intelligence are neural networks, machine learning, and data science.
Neural networks
The human brain is designed to act and interact in accordance with a set of data (memories, actions, rules, norm, traditions, education, religion, politics) received from the environment. This data actually constitutes a unique individual, affecting his career and opportunities. Artificial neural networks is a technology based on studies of the brain and nervous system. These networks imitate a biological neural network, but use an abridged set of concepts of biological neural network. In particular, ANN models imitate the electrical activity of the brain and nervous system. Processing elements (also known as neurodes or perceptrons) are connected to other elements. Neurodes are normally located in a layer or vector with the output of one layer as an input signal for the next layer and possibly other layers. The neurod can be connected with all or a subset of neurons in the next layer and these compounds imitate synaptic connections of the brain. The signals of data coming into the neurode imitate the electrical exications of the nerve cell and, therefore, the transmission of information within a network or brain. The input values for the processing element are multiplied by the weight of the connection and that imitate strengthening of neural pathways in the brain. Learning is emulated in ANN precisely by adjusting the strength or weight of the connection.
Artificial neural networks are used to model the work of the human brain. Artificial neural networks are built like a human brain with nodes of neurodes connected to each other. They are a significant part of computer systems designed to imitate how the human brain analyses and processes information.
In the work “Artificial Intelligence: a Modern Approach” written by Stuart Russell and Peter Norvig2, the emphasis is on intellectual agents. The authors assert that artificial intelligence is aimed at "studying agents, who receive information from the environment and perform actions based on its analysis." The focus is especially on the analysis of rational agents, which work in order to achieve the best results, noting that: "all the skills needed for the Turing test also allow the agent to act rationally."
Stuart Russell and Peter Norvig investigate four different approaches that have historically been defined in the field of artificial intelligence:
- Thinking in a human way
- Acting in a human way
- Thinking rationally
- Act rationally
The first two ideas regard the processes of thinking and the other concern behaviour.
Neural networks are a set of algorithms that attempt to recognize patterns, connections and information from data, the processes of the human brain.
Researchers of artificial intelligence develop the prerequisite of consciousness and then teach the machine to imitate it. Patrick Winston, a professor of artificial intelligence and computer science at the Massachusetts Institute of Technology, defines artificial intelligence as "algorithms enabled by constraints, exposed by representations that support models targeted at loops that tie thinking, perception and action together."3
3 PRESENTATION OF THE PORTRAIT OF PATRICK HENRY WINSTON TO THE SUPREME COURT OF NORTH CAROLINA
Machine learning is a branch of artificial intelligence that allows software applications to be more accurate in predicting results without being explicitly programmed to do so. Machine learning algorithms use historical data as input data to predict new output values.
Classical machine learning is classified according to how the algorithm learns to become more accurate in its predictions. There are four main approaches: supervised learning, unsupervised learning, semi-supervised learning, and reinforcement learning. The type of algorithm chosen by data scientists depends on the type of data they want to predict.
Supervised learning. In this type of machine learning data scientists provide algorithms with labelled learning data and determine the variables they want the algorithm to evaluate for correlation. Both the input and output are indicated.
Unsupervised learning. This type of machine learning is based on algorithms that use unlabelled data. Datasets are scanned and a meaningful connection is searched. The data used to train algorithms, to generate predictions or recommendations are determined beforehand.
Semi-supervised learning is an approach to machine learning that combines the two previous types and uses algorithms based on the selected data, but such a model facilitates their independent study and generates particular understanding of the dataset.
Reinforcement learning is an approach used to acquire skills of performing multi-stage processes according to clearly defined rules. The algorithm of task execution with submission of positive or negative signals is programmed. The algorithm facilitates decision-making regarding the sequence of steps.
Reinforcement learning provides the ability to use the algorithm with both labelled inputs and desired outputs. Controlled machine learning algorithms are used to perform the following tasks:
- Binary classification: the division of data into two categories.
- Multi-class classification: choosing between several options.
- Regression modelling: predicting continuing values.
- Ensembling: a combination of predictions made using machine learning approaches.
Thus, machine learning is a set of methods, a set of algorithms that can be used to create a machine that is able to learn from its own experience and can process huge amounts of input data, find patterns in them in order to generate recommendations for decision-making based on the obtained data.
The article “Introduction to Deep Learning” analyses the usage of a simple neural network to build machine learning packages that provide data processing, modelling, evaluation and optimization. Programs learn from existing data and apply this knowledge to new data or use it to make predictions. The ability of artificial intelligence to interact with humans and the environment is subjected to general rules. The possibility of using deep learning to train artificial intelligence to act with a certain degree of self-consciousness according to the situation is being considered. Artificial intelligence systems are designed to imitate the human brain’s work. Artificial neurons are organised in layers that provide processing of the information. In recent years, deep learning technologies have undergone significant improvements, which contributes to their usage in order to perform tasks in various fields.
Artificial intelligence systems are adapted by using progressive learning algorithms so that data can perform programming. Artificial intelligence finds structure and patterns in data in order for algorithms to acquire skills. Just as an algorithm can teach itself to play chess, it can learn which product to recommend the next online. So, models adapt when they receive new data. Artificial intelligence achieves incredible accuracy due to deep neural networks. Artificial intelligence gets the most out of data. The data itself is an asset in the process of algorithm’s self-learning.
Data Science is a science of data analysis methods in order to obtain valuable information from them.
90% of all data in the world was created in the last two years. Not only does not the process of data generation stop, but also it is stated that its amount has reached 2.7 zettabytes and in the coming years it is going to increase to 44 zettabytes. The amount of big data generated by nowadays’ digital economy grows at a rate of 40% each year and is expected to reach 163 trillion gigabytes by 2025. Such an increase in data stimulates the improvements of artificial intelligence algorithms.
This industry is developing so fast and revolutionising so many other industries that it is difficult to limit it with a formal definition. Data science is designed to extract information from raw data to achieve practical goals. The current wave of development of the artificial intelligence industry is because of both the availability of big data and the improvements of software and hardware.
Modern digital data is essential for the functioning of business, conducting diverse research and just for daily life. Data scientists have become a necessary asset over the last decade and are now working in almost all organisations. These professionals contribute to data management, have high-quality technical skills and are able to build complex quantitative algorithms for processing and synthesis of vast amounts of information used in order to shape strategies in its organisation.
Data science generates new products that promote breakthrough ideas by analysing large amounts of data and searching for connections and patterns. The term "data scientist" appeared in 2008 when companies realised their need for data professionals who know how to organise and analyse huge amounts of data. Hal Varian, Google’s chief economist and professor of information science, business and economics at the University of California, Berkeley, highlighted the significance of adapting to the impact of technology and reconfiguring different industries: “The ability to take data - to be able to understand it, to process it, to extract value from it, to visualizate it, to communicate it - that is going to be hugely important skill in the next decades.”4
4 Hal Varian, The McKinsey Quarterly, January 2009
Effective data scientists can identify relevant issues, collect data from a wide variety of sources, organise them, convert results into solutions and communicate their conclusions in such a way that positively influences business decisions. These skills are needed in almost all fields, so qualified data scientists are becoming increasingly valuable for companies. Data scientists should be result-oriented, have exceptional industry knowledge and communication skills that allow them to explain high tech results to their colleagues, who have no technical knowledge.
Data scientists must be experts in a variety of fields, including data engineering, mathematics, statistics and information technology in order to be able to effectively analyse vast amounts of unstructured information and identify critical elements that can help to stimulate the development of innovation.
Data scientists rely on artificial intelligence to great extent, especially in areas such as machine learning and deep learning in order to create models and make predictions using diverse tools and techniques.
The usage of data science. Companies hire data scientists with the aim to obtain, manage and analyse large amounts of unstructured data. The results are then synthesised and communicated to key stakeholders for strategic decision-making in the organisation. Data analysts bridge the gap between data scientists and business analysts. They are asked different questions that the organisation needs to be answered and then they organise and analyse the data to find the appropriate for the high-level business strategy results. Data analysts are responsible for converting technical analysis into qualitative actions and effective communication their conclusions to stakeholders.
Data science assists in achievement of essential goals, which used to be completely impossible or required much more efforts only a few years ago, such as:
- Detection of anomalies (fraud, disease, crime, etc.)
- Automation of decision making (verification of data, determination of solvency, etc.)
- Classifications (for instance, on the email server that is classifying of emails as ‘important” or “junk”)
- Predictions (sales, profits and customer retention)
- Identification of patterns (weather conditions, financial market models, etc.)
- Recognition (face, voice, text, etc.)
- Recommendations (based on learned preferences, recommendation systems can lead to movies, restaurants, and books a person may like)
The number of examples of the companies’ usage of data science to innovate their sectors, create new products and improve the efficiency of the world around them can be endless. Data science has led to breakthroughs in the healthcare industry. Due to the usage of big data processing technologies, the sources of which are clinical databases and personal fitness trackers, medicine workers received new tools for diagnosing disease, tacking preventing measurements and forming new treatment options.
Tesla, Ford and Volkswagen are implementing predictive analytics in a new generation of autonomous vehicles - self-driving cars. These cars use thousands of cameras and sensors for transmitting information in real time. Unmanned vehicles can adapt to speed limits, avoid dangerous lane changes and carry passengers on the fastest route by using machine learning, predictive analytics and data science.
The logistics business uses data science to maximise the efficiency of delivery routes. The developed convenient tool of integrated optimization and navigation on the road is based on statistical modelling and algorithms, which are based on data on weather conditions, traffic etc. Researchers assert that data science saves the logistics company up to 39 million gallons of fuel and the need to overcome more than 100 million miles of delivery each year.
The life cycle of data science can be characterised by the following five stages:
- Obtaining data (data retrieval, data entry, signal reception, data extraction)
- Data maintenance (data storage, data cleaning, data production, data architecture)
- Data processing (data mining, clustering/classification, data modelling, data consolidation)
- Data analysis (exploratory/confirmatory, predictive analysis, regression, text analysis, qualitative analysis)
- Presenting the results (data reporting, data visualisation, business analytics, decision making)
The future will definitely be robotic owing to the usage of artificial intelligence. Robots will become an essential part of personal and professional life. The most modern type of robots are humanoids, who are the professional service robots created to imitate human emotions and actions. They are becoming commercially viable in a wide range of applications.
The market of humanoid robots is ready for significant growth. This market is anticipated to be valued at $ 3.9 billion in 2023, growing by a staggering 52.1% by 2023. The rapid expansion of the humanoid robots market is primarily due to the rapid improvements of capabilities of these robots and their viability in constantly wider range of applications.
Humanoid robots are commonly used to perform time consuming and dangerous tasks. The wide range of tasks from dangerous rescue to compassionate care can be automated by humanoid robots. The methods of development of these robots are constantly expanding and, as the basic technologies improve, the market will develop intensively.
An ecosystem of United Robotics Group experts and partners has been created in order to join forces to create hardware, software and know-how for the best robotics solutions.
A presentation of the humanoid robot Sophia, which is the latest and most advanced robot using artificial intelligence of the Hong Kong-based company Hanson Robotics, was held in 2016. Sophia became a media-sensation by giving numerous interviews, performing at concerts and being even on the cover of one of the leading fashion magazines.
Hanson Robotics is going to mass produce four robot models, including Sophia, in anticipation of the new opportunities for the robotics industry that will be provided by the pandemic.
Hanson considers the robotics solutions to deal with the pandemic not only to be not limited to healthcare, but also to assist customers in industries such as retail and airlines
Social robotics professor Johan Hoorn, whose research has included work with Sophia, asserted that although the technology is still in relative infancy, the pandemic could accelerate a relationship between humans and robots.
Pepper robot from SoftBank robotics was used in order to identify people who do not wear masks. China-based robotics company CloudMinds assisted in building a field hospital during a coronavirus outbreak in Wuhan.
Researchers assert that artificial intelligence solutions bring the digital society to the higher level.